AI in Cybersecurity: The Role of Artificial Intelligence in Combating Cyber Threats
In the digital age, cybersecurity is not just a necessity but a crucial battleground where Artificial Intelligence (AI) is becoming an invaluable ally. As cyber threats evolve in complexity and sophistication, traditional security measures struggle to keep pace. AI is transforming the field by enabling more proactive, adaptive, and robust cybersecurity defenses. This comprehensive exploration delves into how AI enhances threat detection, analysis, and response, ensuring businesses can fend off even the most advanced cyber threats.
The Evolution of AI in Cybersecurity
Artificial Intelligence has gradually transitioned from experimental applications to a cornerstone of modern cybersecurity strategies. Initially utilized for basic tasks such as scanning for viruses and malware, AI has now evolved to handle more complex duties including real-time threat detection, predictive analytics, and automated incident response. This evolution reflects the growing need for systems that can learn from past attacks to better predict and mitigate future threats.
AI-Driven Threat Detection & Real-World Applications
In the realm of cybersecurity, AI-driven threat detection has proven instrumental in identifying and neutralizing potential threats before they can cause harm. Here, we delve into real-world examples to illustrate the capabilities and effectiveness of AI in this critical area.
Case Study 1: Detecting Advanced Persistent Threats (APTs)
Advanced Persistent Threats (APTs) represent a category of cyber attacks that are continuously evolving and can remain hidden within a network for an extended period. AI systems are particularly adept at detecting these because they can analyze large volumes of network data in real-time, identifying subtle patterns that indicate the presence of APTs. For instance, an AI system at a major financial institution successfully identified irregular data transmissions that were traced back to a stealthy infiltration by an APT. The AI system’s ability to correlate disparate data points across the network allowed for early detection and prevention of substantial data loss.
Case Study 2: Phishing Detection
Phishing attacks are increasingly sophisticated, often bypassing traditional spam filters. AI has transformed phishing detection by analyzing the content of emails and their metadata for signs that they might be part of a phishing attempt. For example, an AI-powered email filtering system implemented by a global retail company was able to reduce phishing incidents by over 60% in the first six months. The system used natural language processing to detect subtle cues in language and structure that were indicative of phishing, significantly improving the security posture of the company.
Case Study 3: Real-time Malware Detection
Malware can compromise a network within minutes of infiltration, making real-time detection crucial. AI systems, equipped with machine learning, can detect malware at the point of entry by analyzing the behavior of files and applications in real-time. A notable example involved a technology firm where AI detected an unknown malware variant trying to encrypt files for a ransomware attack. By recognizing the abnormal behavior before any files were encrypted, the AI system prevented a potentially devastating attack.
Technological Underpinnings
The technological backbone of AI in threat detection typically involves:
- Machine Learning Algorithms: These algorithms learn from historical cybersecurity incident data to detect patterns and anomalies in network traffic or user behavior that may signify a threat.
- Anomaly Detection Systems: By establishing what normal traffic and behavior look like, these systems can immediately flag activities that deviate from the norm, which are often indicative of cybersecurity threats.
Enhanced Threat Analysis with AI
AI’s capability extends beyond mere detection to a comprehensive analysis of cyber threats. This analysis is crucial for understanding the nature and potential impact of threats, enabling precise and informed decision-making in response strategies. Let’s delve into how AI enhances threat analysis through advanced techniques and real-world applications.
Deep Learning for Complex Threat Patterns
Deep learning, a subset of machine learning, is particularly effective at identifying and understanding complex patterns in data that traditional methods might miss. By using neural networks, AI systems can analyze vast amounts of data from various sources, such as network traffic, user behavior, and previous security incidents, to identify subtle correlations that indicate malicious activity.
For example, in a scenario involving a multinational corporation, AI was used to analyze irregular network traffic patterns that initially appeared benign. The AI system, employing deep learning, discerned that these patterns were indicative of data exfiltration by an external actor. This early identification enabled the company to thwart a potentially severe data breach, safeguarding sensitive information.
Behavioral Analysis for Insider Threats
AI excels in behavioral analysis, which is instrumental in detecting insider threats—a significant and often overlooked risk. AI systems monitor user activities continuously and compare them against established behavioral baselines. Any deviations from these baselines can trigger alerts for further investigation.
A notable application was seen in a government agency where AI was implemented to safeguard against leaks of classified information. The AI system flagged unusual access patterns by a trusted insider, who was subsequently found to be uploading sensitive data to unauthorized cloud storage services. This proactive detection prevented a major security incident.
Predictive Analytics in Threat Intelligence
AI also plays a vital role in predictive analytics, using historical data to predict future security threats and trends. This forward-looking approach allows organizations to prepare and mitigate risks before they become imminent threats.
An example of this is a healthcare provider using AI to predict phishing attacks. By analyzing previous incidents and external intelligence feeds, the AI system could identify likely targets and methods for upcoming attacks, allowing the organization to strengthen its defenses in vulnerable areas proactively.
AI’s Role in Automated Incident Response
Following threat analysis, AI’s capabilities extend to orchestrating automated incident responses. For instance, upon detecting a ransomware attack, an AI system can not only isolate the affected segment of the network but also initiate automated backups and inform response teams with detailed analysis reports, reducing downtime and enhancing recovery processes.
The enhanced threat analysis capabilities of AI transform how organizations approach cybersecurity. With the power to analyze, understand, and predict threats, AI provides a dynamic defense mechanism that adapts and evolves with the threat landscape. This adaptability is crucial for maintaining robust security in an era where cyber threats are continually changing and increasing in sophistication.
Automated Response to Security Incidents
The integration of Artificial Intelligence in cybersecurity goes beyond detection and analysis; AI is pivotal in automating responses to security incidents, enabling rapid containment and mitigation of threats. This automation plays a critical role in minimizing damage and streamlining the incident response process, allowing for a swift and effective resolution.
Real-time Incident Containment and Mitigation
AI’s capability to execute real-time responses to identified threats is a game-changer for cybersecurity. For instance, upon detecting a network intrusion, AI systems can automatically block the IP addresses involved and isolate affected network segments without human intervention. This immediate response prevents the spread of the intrusion and limits potential damage. A practical example of this occurred at a large financial institution where AI systems detected an anomaly suggesting a breach attempt. The AI automatically redirected the traffic to a quarantine zone, analyzed the threat, and applied necessary patches to vulnerable systems—all within minutes of the initial detection.
Scenario-Based Response Strategies
AI systems can be programmed with scenario-based strategies to handle various types of cyber threats. For instance, in the case of ransomware detection, AI can initiate a series of predetermined actions such as shutting down vulnerable systems, initiating backups, and notifying the cybersecurity response team with detailed information about the attack vector and affected data. These actions not only mitigate the immediate threat but also facilitate a quicker recovery. An example of such an application was seen in a tech company where AI-driven systems detected ransomware activity and immediately executed a series of countermeasures that successfully minimized data loss and system downtime.
Enhancing Human Capabilities
While AI significantly automates responses, it also enhances the capabilities of human security teams by providing them with actionable insights and freeing them up from routine tasks to focus on more complex strategic security issues. AI tools supply security teams with a comprehensive analysis of the incident, suggested mitigation strategies, and predictive insights on possible future attacks. This synergy between human expertise and AI automation fosters a more resilient cybersecurity posture, adept at dealing with both current and emerging threats.
The automation of incident response powered by AI not only bolsters security defenses but also ensures that organizations can quickly adapt to and recover from cyber threats. This dynamic capability of AI in automating responses, combined with its predictive and analytical prowess, establishes a robust foundation for any modern cybersecurity strategy.
Challenges and Ethical Considerations of AI in Cybersecurity
Implementing AI in cybersecurity presents several challenges and ethical considerations that organizations must navigate carefully. The integration of AI technologies raises concerns about data privacy, potential biases in decision-making processes, and the overarching implications for individual freedoms and security.
Data Privacy and Security Concerns
AI systems require access to extensive datasets to learn and make informed decisions. This dependency on vast amounts of data poses significant privacy concerns, especially when handling sensitive information. Ensuring that AI systems comply with data protection regulations, such as GDPR in Europe, is crucial. Organizations must implement robust data governance practices to maintain the integrity and confidentiality of the data, mitigating the risk of breaches that could expose sensitive information to malicious actors.
Bias and Fairness in AI Algorithms
Another significant challenge is the potential for bias in AI algorithms, which can lead to unfair or ineffective security measures. AI systems learn from data, and if the data is biased, the decisions made by AI will inherently carry those biases. This can result in overlooking certain threats or falsely identifying benign activities as malicious, which could have serious repercussions for individuals and businesses alike. Ensuring the fairness and accuracy of AI involves continuous monitoring and updating of AI models to correct biases and adapt to new threat landscapes.
These challenges highlight the need for a balanced approach to AI implementation in cybersecurity, where technological advancements are matched with strong ethical standards and practices. Addressing these issues is essential not only for maintaining public trust but also for ensuring that AI-driven security measures are just, effective, and aligned with broader societal values.
Future Directions and Innovations in AI for Cybersecurity
As AI continues to evolve, its role in cybersecurity is set to expand, bringing about new innovations that could redefine how security threats are managed and neutralized. The future of AI in cybersecurity is poised to feature more autonomous systems, enhanced predictive capabilities, and deeper integration with other technological innovations.
Toward Autonomous Security Operations
The advancement of AI technologies is steering cybersecurity toward more autonomous operations. Future AI systems are expected to manage much of the routine security monitoring and response tasks without human intervention. This shift will allow cybersecurity professionals to focus on strategic analysis and complex problem-solving. For instance, AI could autonomously update defenses based on real-time threat intelligence, execute security protocols, and even conduct forensic analysis post-incident to prevent future breaches.
Enhanced Predictive Capabilities
With improvements in machine learning algorithms and data analytics, AI’s predictive capabilities are becoming increasingly precise. Future AI systems will not only detect and respond to threats but will also be able to predict and prevent them before they manifest. This proactive approach could include predictive threat modeling that simulates potential attack scenarios to strengthen defenses proactively. For example, AI could analyze trends in cyber-attack strategies across the globe to predict and prepare for similar attacks on local systems.
Integration with Emerging Technologies
AI’s integration with emerging technologies such as quantum computing, blockchain, and IoT devices promises to bolster cybersecurity measures further. Quantum computing, for instance, could enhance AI’s ability to solve complex problems much faster than current technologies, improving the speed and efficiency of threat detection and response. Similarly, blockchain could provide a secure and transparent way to log and monitor AI actions, enhancing the accountability and traceability of automated security measures.
Artificial Intelligence is transforming cybersecurity from a reactive to a proactive discipline, marked by rapid responses, advanced threat detection, and predictive capabilities. As AI technologies advance, they will become integral to developing robust, dynamic, and adaptive cybersecurity strategies that not only respond to threats but anticipate and neutralize them. For businesses and cybersecurity professionals, staying updated with these AI advancements and integrating them into their security strategies will be key to safeguarding their digital assets in the increasingly complex cyber threat landscape.
Embrace the future of cybersecurity with AI-driven solutions. Schedule a call with a DysrupIT expert to discuss your specific needs and explore the opportunities AI can offer for enhancing your cybersecurity strategy. Visit our blog for the latest insights and innovations in AI for cybersecurity.
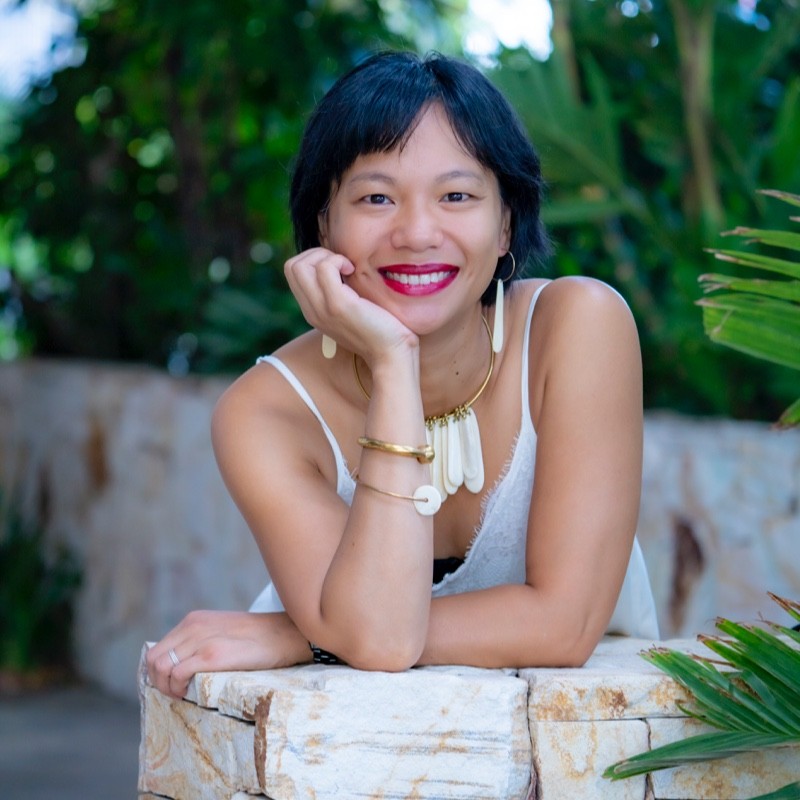
With an impressive professional background in cybersecurity, Elizabeth has held key executive positions throughout Asia-Pacific and Japan. Her extensive experience has played a pivotal role in the exceptional growth and success of DysrupITâ„¢. Her invaluable expertise has transformed the company into a thriving multi-million-dollar global enterprise.